Privacy-Preserving Data Publishing - Part 1: Understanding k-Anonymity
k-Anonymity is a fundamental privacy concept that helps protect individual privacy in datasets. Let's understand how it works.
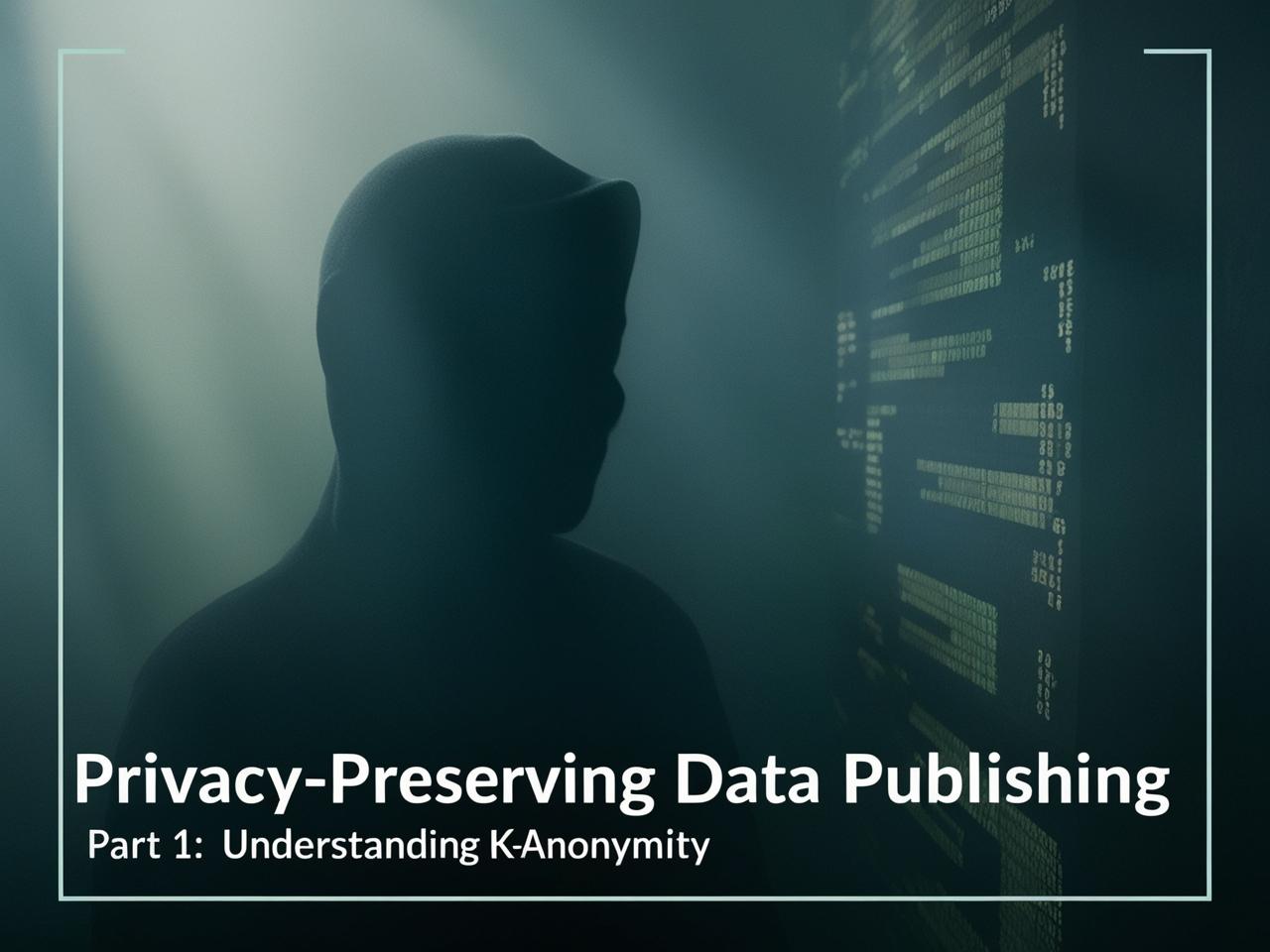
What is k-Anonymity?
k-Anonymity is a privacy model that ensures each record in a dataset is indistinguishable from at least k-1 other records with respect to certain identifying attributes.
Key Concepts
- Quasi-identifiers
- Attributes that could potentially identify an individual
- Examples: age, zipcode, gender
- Must be carefully selected based on dataset context
- Sensitive attributes
- Information that should be protected
- Examples: medical conditions, salary
- Maintained in original form while protecting privacy
- k value
- Determines privacy strength
- Higher k means better privacy but less data utility
- Typically ranges from 3 to 10 in practice
Why is k-Anonymity Important?
Real-world Privacy Breaches
In 1997, the Massachusetts Group Insurance Commission (GIC) released “anonymized” data about state employees’ hospital visits, believing they had protected patient privacy by removing explicit identifiers. However, Dr. Latanya Sweeney demonstrated that by combining this data with publicly available voter registration records, it was possible to uniquely identify Governor William Weld’s medical records. This landmark case highlighted how combining supposedly anonymous data with other public datasets could lead to re-identification.
Source: This case was documented in “k-Anonymity: A Model for Protecting Privacy” by Latanya Sweeney, published in the International Journal of Uncertainty, Fuzziness and Knowledge-Based Systems (2002).
Benefits of k-Anonymity
[Rest of the content remains the same…]
Benefits of k-Anonymity
- Protects against re-identification attacks
- Maintains data utility for analysis
- Provides measurable privacy guarantees
- Supports regulatory compliance
Implementing k-Anonymity
Step 1: Identify Quasi-identifiers
-- Example table structure
CREATE TABLE PatientData (
ID INT,
Age INT, -- quasi-identifier
Zipcode VARCHAR(5), -- quasi-identifier
Gender CHAR(1), -- quasi-identifier
Disease VARCHAR(50) -- sensitive attribute
);
Step 2: Apply Generalization
def generalize_age(age):
if age < 20:
return "0-19"
elif age < 40:
return "20-39"
elif age < 60:
return "40-59"
else:
return "60+"
Step 3: Verify k-Anonymity
-- Check if k-anonymity is satisfied (k=3)
SELECT Age_Group, Zipcode_Group, Gender, COUNT(*)
FROM Anonymized_PatientData
GROUP BY Age_Group, Zipcode_Group, Gender
HAVING COUNT(*) < 3;
Common Pitfalls
- Overlooking quasi-identifiers
- Incomplete identification leads to privacy breaches
- Regular assessment needed as external data changes
- Excessive generalization
- Over-anonymization reduces data utility
- Balance needed between privacy and usefulness
- Small dataset challenges
- Difficult to achieve k-anonymity with limited records
- May require higher generalization levels
Best Practices
- Start with threat modeling
- Identify potential attackers
- Understand available external data
- Define privacy requirements
- Document assumptions
- Record quasi-identifier selection reasoning
- Document generalization hierarchies
- Note k-value justification
- Regular review
- Assess privacy requirements periodically
- Update generalization strategies
- Monitor external data landscape
Next Steps
In Part 2, we’ll explore the Mondrian algorithm, an efficient method for achieving k-anonymity through multi-dimensional partitioning.
Preview of Coming Topics
- Mondrian algorithm implementation
- Optimization techniques
- Performance considerations
- Real-world case studies